Steps to run the demo
Install BangDB
There are many ways to do it:
- Checkout download page.
- Or check releases at BangDB Github repo.
- You may checkout the Github repo as well.
Cd to the usecases/iot-car-sensor-analysis
cd usecases/iot_vehicle_monitoring
After that, copy bangdb-server-2.0 into the server folder
Run the server, agent and cli
cd server
./bangdb-server-2.0 -c hybrid -w 18080> cd ..
cd agent
./bangdb-agent-2.0> cd ..
cd cli
./bangdb-cli-2.0
When you run the cli, you will get a command prompt like following:
server [ 127.0.0.1 : 10101 ] is master with repl = OFF
__ _ _ _ ____ ___ ___
| \ / \ | \ | | | ___\ | \ | \
| / / \ | \| | | | __ | | | /
| \/ ___ \| | \ | | |__||| | | \
|___/_/ \_|_| |_| |_____||___/ |___/
command line tool for db+stream+ai+graph
please type 'help' to get more info, 'quit' or 'exit' to return
Register the schema
schema is a set of streams and processing/actions that could be done/taken as event arrives.
To register the schema:
register schema car_sensor_schema.txt
Train battery_voltage_model
Command train model, initiates a workflow and cli will ask several questions and finally schedules the training, see below to get the details:
train model battery_voltage_model
To register the schema:
what's the name of the schema for which you wish to train the model?: cars
do you wish to read earlier saved ml schema for editing/adding? [ yes | no ]:
BangDB supports following algorithm, pls select from these
Classification (1) | Regression (2) | Lin-regression/Classification (3) | Kmeans (4) | Custom (5)
| IE - ontology (6) | IE - NER (7) | IE - Sentiment (8) | IE - KB (9) | TS - Forecast (10)
| DL - resnet (11) | DL - lenet (12) | DL - face detection (13) | DL - shape detection (14) | SL - object detection (15)
what's the algo would you like to use (or Enter for default (1)): 10
do you wish to select details of algo and params for the forecast model training? [ yes | no ]: yes
For forecast, pls select algo from these
Regression (2) | Lin-regression/Classification (3))
what's the algo would you like to use (or Enter for default (2)): 3
available solver type for libliner
L2R_LR, L2R_L2LOSS_SVC_DUAL, L2R_L2LOSS_SVC, L2R_L1LOSS_SVC_DUAL, MCSVM_CS,
1R_L2LOSS_SVC, L1R_LR, L2R_LR_DUAL, L2R_L2LOSS_SVR = 11, L2R_L2LOSS_SVR_DUAL, L2R_L1LOSS_SVR_DUAL
tuning of params is only available for solver L2R_LR (0), L2R_L2LOSS_SVC (2) and L2R_L2LOSS_SVR (11)
solver type [ 0 | 1 | 2 | 3 | 4 | 5 | 6 | 7 | 11 | 12 | 13 ] (press enter for default (0)): 11
enter C (or press enter for default):
enter nr_weight (or press enter for default(1)):
enter p, epslon_svr (or press enter for default(0.1)):
enter bias (or press enter for default (0)):
enter fold (or press enter for default (5)):
what's the stopping criteria (or press enter for default (0.001)):
what's the format of the file [ CSV (1) | JSON (2) ] (or Enter for default (1)): 1
enter the time-stamp field name: time
enter the time-stamp field datatype [ string (5) | long (9) | double (11) ] (or Enter for default (9)): 9
what's the position of timestamp field in the csv (count starts with 0): 0
do you wish to use entity for groupby? [ yes | no ]: yes
enter the entity (group by) field name: car
enter the entity (group by) field datatype [ string (5) | long (9) | double (11) ] (or Enter for default (9)): 9
what's the position of entity (group by) field in the csv (count starts with 0, enter -1 ignore): 1
enter the quantity field name: battery_voltage
what's the position of quantity field in the csv (count starts with 0): 6
do you wish to ignore grouping by entity? [ yes | no ]:
what's the granularity [ day (1) | week (2) | month (3) | year (4) | no_rollup(5) ] for aggregation during rollup of quantity (default is day(1)): 5
what's the lag for data to be selected for prediction (default is 2)?: 5
what's the offset for data to be selected for prediction (default is 0)?: 0
do you wish to schedule for the training of the forecast model now? [ yes | no ]: yes
You can check the status of model by typing
show models
Ingest the data (from train.txt file)
cd ../mon bash sendfile ../data/train.txt car.txt 100 1 cd ../cli
The above command will simulate 100 cars sending data every second. Wait until it sends 7000 events.
Train model speed_pred_model
This will predict the speed of the car
train model speed_pred_model
do you wish to read earlier saved ml schema for editing/adding? [ yes | no ]:
BangDB supports following algorithm, pls select from these
Classification (1) | Regression (2) | Lin-regression/Classification (3) | Kmeans (4) | Custom (5)
| IE - ontology (6) | IE - NER (7) | IE - Sentiment (8) | IE - KB (9) | TS - Forecast (10)
| DL - resnet (11) | DL - lenet (12) | DL - face detection (13) | DL - shape detection (14) | SL - object detection (15)
what's the algo would you like to use (or Enter for default (1)): 3
available solver type for libliner
L2R_LR, L2R_L2LOSS_SVC_DUAL, L2R_L2LOSS_SVC, L2R_L1LOSS_SVC_DUAL, MCSVM_CS,
1R_L2LOSS_SVC, L1R_LR, L2R_LR_DUAL, L2R_L2LOSS_SVR = 11, L2R_L2LOSS_SVR_DUAL, L2R_L1LOSS_SVR_DUAL
tuning of params is only available for solver L2R_LR (0), L2R_L2LOSS_SVC (2) and L2R_L2LOSS_SVR (11)
solver type [ 0 | 1 | 2 | 3 | 4 | 5 | 6 | 7 | 11 | 12 | 13 ] (press enter for default (0)): 11
enter C (or press enter for default):
enter nr_weight (or press enter for default(1)):
enter p, epslon_svr (or press enter for default(0.1)):
enter bias (or press enter for default (0)):
enter fold (or press enter for default (5)):
what's the stopping criteria (or press enter for default (0.001)):
what's the input (training data) source? [ local file (1) | file on BRS (2) | stream (3) ] (press enter for default (1)): 3
enter the name of the stream from which the data should be uploaded: sensor
enter the approx of the size of the file in MB(enter for default): 10
enter the duration is seconds upto which the data will be selected from the stream for training (enter for default 3600 sec):
what is the target index (to select the target attribute/val): 0
what's the training speed you wish to select [ Very fast (1) | fast (2) | medium (3) | slow (4) | very slow (5) ] (or Enter for default (1)): 3
what's the attribute type [ NUM (1) | STRING (2) | HYBRID (3) ] (press enter for default (1):
do you wish to scale the data? [ yes | no ]: no
do you wish to tune the params? [ yes | no ]: yes
We need to do the mapping so it can be used on streams later. This means we need to provide attr name and its position in the training file.
attr name: speed
attr position: 0
do you wish to add more attributes? [ yes | no ]: yes
attr name: coolant_temp
attr position: 1
do you wish to add more attributes? [ yes | no ]: yes
attr name: intake_air_temp
attr position: 2
do you wish to add more attributes? [ yes | no ]: yes
attr name: intake_air_flow_speed
attr position: 3
do you wish to add more attributes? [ yes | no ]: yes
attr name: battery_percentage
attr position: 4
do you wish to add more attributes? [ yes | no ]: yes
attr name: battery_voltage
attr position: 5
do you wish to add more attributes? [ yes | no ]: yes
attr name: current_draw
attr position: 6
do you wish to add more attributes? [ yes | no ]: yes
attr name: engine_vibration_amplitude
attr position: 7
do you wish to add more attributes? [ yes | no ]: yes
attr name: throttle_pos
attr position: 8
do you wish to add more attributes? [ yes | no ]:
do you wish to add external udf to do some computations before the training? [ yes | no ]:
do you wish to start training now? [ yes | no ]: yes
Ycan check the status of the model by typing
show models
+--------------------------+---------------------+----+------------+-----------+------------------------+------------------------+
|key |model name |algo|train status|schema name|train start time |train end time |
+--------------------------+---------------------+----+------------+-----------+------------------------+------------------------+
|cars:speed_pred_model |speed_pred_model | LIN|passed |cars |Mon Dec 13 11:28:38 2022|Mon Dec 13 11:29:12 2022|
+--------------------------+---------------------+----+------------+-----------+------------------------+------------------------+
|cars:battery_voltage_model|battery_voltage_model| LIN|passed |cars |Mon Dec 13 11:15:26 2022|Mon Dec 13 11:15:59 2022|
+--------------------------+---------------------+----+------------+-----------+------------------------+------------------------+
Now start ingesting data from other file, this time ingest slowly to see some results.
cd ../mon bash sendfile ../data/test.txt car.txt 10 1 cd ../cli
To see summary of events in different streams
select stream from cars
To select data from sensors stream
select * from cars.sensor
To see aggregated data for coolant_temp
select aggr(coolant_temp) from cars.sensor rollup 0
You can also plot the data in the terminal
select * from cars.sensor where _pk > 1 plot {"type":"line","attrs":["speed","speed_pred"],"just_plot":1} limit 25
bangdb_plot scan
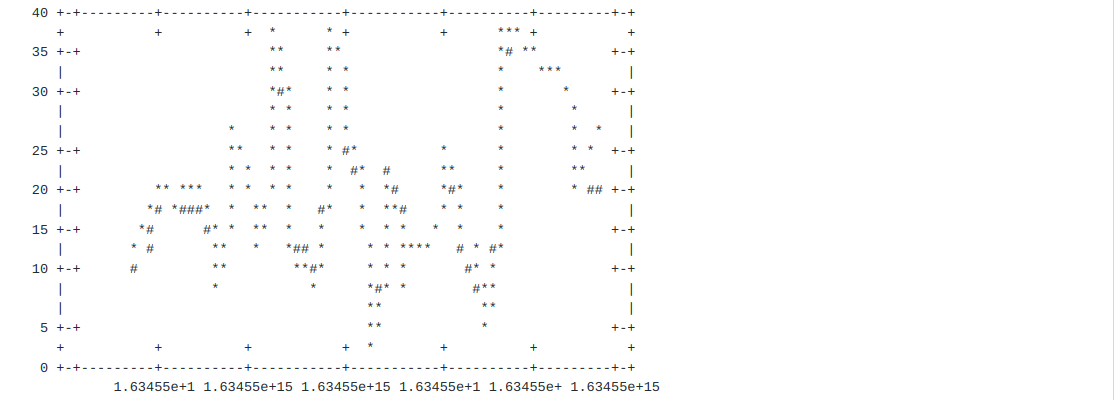
total rows retrieved = 25 (25)
more data to come, continue .... [y/n]: n
To similate the pattern
Insert following events (cut and paste on the cli prompt one by one)
insert into cars.sensor values null
{
"time":1567607187,
"car":100,
"coolant_temp":191.3604,
"intake_air_temp":19.891075,
"intake_air_flow_speed":9.86402,
"battery_percentage":-18.83759,
"battery_voltage":242.07639,
"current_draw":15.151241,
"speed":35.703476,
"engine_vibration_amplitude":2570.3477,
"throttle_pos":0.6609447000000001,
"tire_pressure_1_1":22,
"tire_pressure_1_2":34,
"tire_pressure_2_1":31,
"tire_pressure_2_2":33,
"accelerometer_1_1_value":0.0812409499999999,
"accelerometer_1_2_value":3.3291862,
"accelerometer_2_1_value":3.0881116,
"accelerometer_2_2_value":0.033119857,
"control_unit_firmware":1000
}
insert into cars.sensor values null
{
"time":1567607187,
"car":100,
"coolant_temp":191.3604,
"intake_air_temp":19.891075,
"intake_air_flow_speed":9.86402,
"battery_percentage":-18.83759,
"battery_voltage":242.07639,
"current_draw":15.151241,
"speed":36.703476,
"engine_vibration_amplitude":2570.3477,
"throttle_pos":0.6609447000000001,
"tire_pressure_1_1":22,
"tire_pressure_1_2":34,
"tire_pressure_2_1":31,
"tire_pressure_2_2":33,
"accelerometer_1_1_value":0.0812409499999999,
"accelerometer_1_2_value":3.3291862,
"accelerometer_2_1_value":3.0881116,
"accelerometer_2_2_value":0.033119857,
"control_unit_firmware":1000
}
insert into cars.sensor values null
{
"time":1567607187,
"car":100,
"coolant_temp":191.3604,
"intake_air_temp":19.891075,
"intake_air_flow_speed":9.86402,
"battery_percentage":-18.83759,
"battery_voltage":242.07639,
"current_draw":15.151241,
"speed":37.703476,
"engine_vibration_amplitude":2570.3477,
"throttle_pos":0.6609447000000001,
"tire_pressure_1_1":22,
"tire_pressure_1_2":34,
"tire_pressure_2_1":31,
"tire_pressure_2_2":33,
"accelerometer_1_1_value":0.0812409499999999,
"accelerometer_1_2_value":3.3291862,
"accelerometer_2_1_value":3.0881116,
"accelerometer_2_2_value":0.033119857,
"control_unit_firmware":1000
}
insert into cars.sensor values null
{
"time":1567607187,
"car":100,
"coolant_temp":191.3604,
"intake_air_temp":19.891075,
"intake_air_flow_speed":9.86402,
"battery_percentage":-18.83759,
"battery_voltage":242.07639,
"current_draw":15.151241,
"speed":38.703476,
"engine_vibration_amplitude":2570.3477,
"throttle_pos":0.6609447000000001,
"tire_pressure_1_1":22,
"tire_pressure_1_2":34,
"tire_pressure_2_1":31,
"tire_pressure_2_2":33,
"accelerometer_1_1_value":0.0812409499999999,
"accelerometer_1_2_value":3.3291862,
"accelerometer_2_1_value":3.0881116,
"accelerometer_2_2_value":0.033119857,
"control_unit_firmware":1000
}
If you run “select stream from cars” you will see one event there in the cep output stream. Basically we simulated continulously increasing speed of a particular car for 3 times in 1000 sec window.
Useful Resources
- Please go to https://bangdb.com/developer for more info on BangDB.
- Please subsribe to our YouTube channel for more videos on different real world use cases.
- Please follow us on twitter at @iqlect